How AI Image Generators Work: A Deep Dive into the Technology

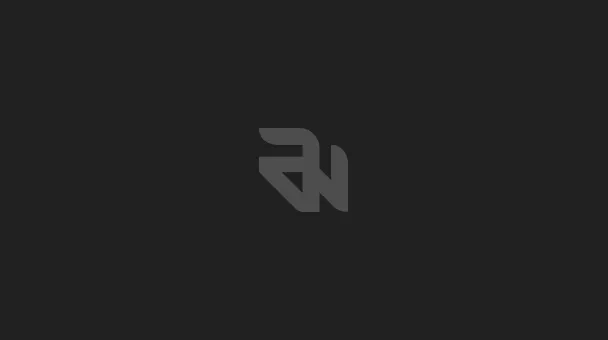
In the rapidly evolving field of artificial intelligence, AI image generators stand out for their remarkable ability to create visually stunning and contextually accurate images from simple text prompts. In this blog, we’ll explore the inner workings of these sophisticated tools, shedding light on the technologies that make them possible. We’ll break down how AI image generators interpret and translate textual descriptions into detailed visuals, offering a glimpse into the future of digital art and design.
We’ll dive into the core technologies driving these systems, including Text Understanding with Natural Language Processing (NLP), Generative Adversarial Networks (GANs), Diffusion Models, and Neural Style Transfer (NST). Each of these techniques plays a crucial role in transforming abstract concepts into concrete imagery, allowing for the creation of everything from realistic portraits to fantastical scenes.
By the end of this blog, you’ll have a comprehensive understanding of how AI image generators work, equipping you with the knowledge to leverage these tools in creative and innovative ways. Whether you’re a tech enthusiast, artist, or designer, this exploration will enhance your grasp of AI’s potential in the visual domain.
What is an AI Image Generator?
An AI image generator is a powerful tool that creates images based on the text or other input you provide. Imagine you describe a scene, like “a sunset over a mountain,” and the AI transforms that description into a visual representation. This technology uses complex algorithms to understand your input and generate images that match your description. It’s incredibly useful for artists, designers, and marketers who need custom visuals quickly.
Instead of spending hours creating or sourcing images, you can generate exactly what you need with just a few words. AI image generators streamline the creative process, allowing for more creativity and efficiency. They can produce a wide range of visuals, from realistic photographs to abstract artwork, making them a versatile tool in many creative and professional settings.
How do AI Image Generators Work?
AI image generators use sophisticated technologies to create detailed and accurate images from various inputs. Four key techniques driving this process are Text Understanding using Natural Language Processing (NLP), Generative Adversarial Networks (GANs), Diffusion Models, and Neural Style Transfer (NST). Text Understanding through NLP translates textual prompts into machine-readable formats, while GANs employ a competitive network structure to generate realistic images.
Diffusion Models create images by iteratively adding and removing noise, and NST combines the content of one image with the style of another. Let’s delve into each of these methods in detail to understand how they contribute to the magic of AI image generation.
1. Text understanding using NLP
Text understanding using Natural Language Processing (NLP) is a crucial component in AI image generation. It involves converting textual prompts into machine-readable formats, which AI systems use to create images. This process begins with NLP models, like CLIP (Contrastive Language-Image Pre-training), which translate text into numerical representations known as embeddings. These embeddings capture the semantic meaning and context of the text.
For instance, if a user inputs "a golden retriever playing in the park," the NLP model encodes this text into high-dimensional vectors that represent the key elements—"golden retriever," "playing," and "park." These vectors guide the image generator to incorporate and position these elements accurately within the generated image.
NLP’s role extends beyond just text-to-image translation; it also helps in refining image details based on the text’s nuances. This technology enables AI systems to understand and visualise complex textual descriptions, making it integral to creating accurate and contextually relevant images.
2. Generative Adversarial Networks (GANs)
Generative Adversarial Networks (GANs) are a key technology in AI for creating images. They work with two parts: a generator and a discriminator. The generator makes fake images while the discriminator tries to tell if the images are real or fake.
The two parts are in a contest. The generator tries to create images that look as real as possible, while the discriminator tries to spot which images are fake. Over time, as they compete, both parties get better at their jobs. The generator makes more convincing images, and the discriminator gets better at spotting fakes.
GANs are used in many areas. They help create realistic images of people, improve video game graphics, and even generate new artwork. Their ability to produce high-quality, lifelike images makes them valuable in many creative and practical applications.
3. Diffusion Models
Diffusion models are a sophisticated AI technique used for generating images. Here's a step-by-step explanation of how they work:
1. Starting Point
In this initial phase, the diffusion model begins with a clear image. This image is progressively altered by adding random noise to it. Think of it like smudging a picture with a blurry, static-like effect. This process is repeated over several steps, making the image increasingly indistinct and blurry. The purpose of this step is to create a variety of noisy images from the original one, effectively learning how noise affects image clarity.
2. Learning Phase
During training, the model focuses on understanding how the added noise transforms the original image. It works through a series of noisy images and their corresponding clear versions. The model's goal is to learn the pattern of noise addition and how to reverse it. By analysing the noisy images and comparing them to the clear originals, the model develops an understanding of how each type of noise affects the image's clarity. This helps the model learn how to remove noise step-by-step to restore the image to its original state. Essentially, the model builds a guide on how to reverse the blurring process effectively.
3. Image Generation
When the model is ready to create a new image, it starts with random noise, similar to the blurry starting point in the initial phase. Using the knowledge acquired during training, the model removes this noise gradually. This is done in multiple stages, with each step refining the image by reducing the noise and adding more detail. The process is guided by the input text or prompt provided by the user. For example, if the prompt is “a serene beach at sunset,” the model uses the prompt to shape the noise into an image that resembles a beach scene with the specified characteristics. The result is a clear, coherent image generated from random noise tailored to match the input prompt.
Diffusion models excel at generating high-quality and realistic images from scratch. They are widely used in art creation, graphic design, and virtual reality, offering a powerful way to produce detailed and visually appealing images.
4. Neural Style Transfer (NST)
Neural Style Transfer (NST) is a technique that combines the content of one image with the artistic style of another to create a new, unique image. Here’s how it works:
1. Content and Style Images
The process starts with two images: a content image and a style image. The content image features the main subject or scene, while the style image provides artistic elements such as textures, colours, and patterns. By combining these two images, Neural Style Transfer aims to blend the visual structure of the content image with the artistic style of the style image.
2. Feature Extraction
A deep learning model, like a Convolutional Neural Network (CNN), extracts features from both images. For the content image, it captures the shapes and structures. The style image identifies textures and colours. This extraction process helps the model understand what makes each image unique.
3. Generating the New Image
Using the features extracted, the model generates a new image that incorporates the content of the content image and the style of the style image. The process involves iterative refinement to ensure the final image effectively balances the original content with the desired artistic style.
NST is widely used to create visually appealing artworks, transform photos into different artistic styles, and generate creative visual content.
Benefits of Using AI Image Generators
AI image generators offer transformative benefits for various creative and professional needs, simplifying the process of creating custom visuals. By leveraging advanced technology, these tools make it possible to produce high-quality images quickly and efficiently. Whether you're an artist, marketer, or designer, AI image generators can significantly enhance your workflow and creative capabilities.
1. Speed and Efficiency
AI image generators drastically reduce the time needed to create visuals. What might take hours or days to design manually can be done in minutes, allowing you to meet tight deadlines and streamline your creative process.
2. Cost-Effectiveness
Utilising AI image generators can save on costs associated with hiring designers or purchasing stock images. With a few simple inputs, you can generate high-quality images without incurring additional expenses, making it a budget-friendly solution.
3. Customization and Flexibility
These tools offer extensive customisation options, enabling you to tailor images to specific needs. Whether you require a particular style or theme, AI generators can adjust and refine images according to your exact specifications.
4. Creative Exploration
AI image generators open up new possibilities for creative experimentation. You can explore various styles and concepts quickly, allowing you to test out ideas and visualise different approaches without committing significant time or resources.
5. High-Quality Output
Advanced AI models are capable of producing images with impressive detail and realism. This high-quality output enhances the visual appeal of your projects, whether for marketing materials, social media, or artistic endeavours.
Challenges and Considerations of AI Image Generators
AI image generators are transformative tools that offer remarkable creative possibilities, but they come with their own set of challenges and considerations. As these technologies evolve, understanding their limitations and ethical implications becomes crucial for users and developers alike. Let's explore key challenges associated with AI image generators, providing insights into their complexities and offering guidance on how to navigate these issues effectively.
1. Bias and Fairness
AI image generators can inadvertently perpetuate biases present in their training data. This may result in images that reinforce stereotypes or exclude certain groups. Ensuring diverse and representative datasets can mitigate these issues and promote fairness in generated content.
2. Intellectual Property Concerns
Using AI to create images based on existing artworks or designs can raise intellectual property issues. Users should be cautious about generating content that closely resembles copyrighted material to avoid legal complications and respect the original creators' rights.
3. Quality and Accuracy
While AI image generators are powerful, they can sometimes produce images that lack detail or accuracy. Users may need to refine prompts and make adjustments to achieve the desired quality, and post-processing might be necessary for polished results.
4. Ethical Use
AI-generated images can be used in misleading or harmful ways, such as creating deepfakes or deceptive advertisements. Users need to adhere to ethical guidelines and ensure that their use of AI-generated content is transparent and responsible.
5. Technical Limitations
AI image generators have technical limitations, such as processing power and model constraints, that can affect performance. Understanding these limitations and managing expectations can help users better utilise the technology and avoid frustration with suboptimal results.
Unveiling BharatDiffusion—The Future of Indian AI Image Generation
What exactly is BharatDiffusion? Imagine an advanced Indian AI image generator that brings the rich and diverse essence of India to life through stunning visuals. BharatDiffusion leverages cutting-edge diffusion technology to create highly realistic and culturally immersive Indian AI images, capturing the vibrant landscapes, traditions, and heritage of India. Ideal for artists, designers, and anyone looking to incorporate traditional AI images into their projects, this innovative tool delivers high-quality, visually engaging results.
What makes BharatDiffusion truly exceptional is its extensive training on a diverse dataset of Indian imagery. This ensures that each image authentically reflects India’s multifaceted culture. From historic landmarks and traditional festivals to modern cityscapes, BharatDiffusion excels in producing captivating AI traditional images that tell a unique story.
Sign up today to be among the first to experience this transformative tool. Once registered, you’ll receive 50 free images to start exploring and incorporating the rich tapestry of Indian culture into your work. Embrace this opportunity to enhance your visual content with BharatDiffusion’s culturally resonant imagery and stand out with authentic Indian AI images.
Conclusion
AI image generators represent a revolutionary advancement in digital art, offering both immense benefits and notable challenges. We've explored how these technologies work, from transforming text prompts into visuals to creating detailed and creative images. While they provide remarkable advantages like efficiency and customisation, they also come with considerations such as quality control and ethical use. Understanding these aspects helps leverage AI image generators effectively while addressing their limitations. As this technology evolves, it continues to reshape the creative landscape with exciting possibilities.